🔗Accurate predictions on small data with a tabular foundation model | Nature
1. Introduction
Tabular data — spreadsheets with rows and columns — is foundational in science, used in fields from biomedicine to climate research. Yet, traditional deep learning models perform poorly on tabular data due to its high heterogeneity. Enter TabPFN: a Tabular Prior-data Fitted Network, a novel foundation model designed for small datasets (up to 10,000 samples). Unlike traditional models that require large datasets and heavy tuning, TabPFN achieves state-of-the-art performance with minimal training and in a fraction of the time. It's built as a generative transformer-based model, enabling tasks such as fine-tuning, density estimation, and reusable embeddings — all by learning to learn across millions of synthetic datasets.
2. Principled In-Context Learning (ICL)
TabPFN borrows the powerful concept of in-context learning from large language models. Instead of being hand-crafted, the algorithm itself is learned by training on a wide variety of synthetic prediction tasks. The process works as follows:
Synthetic Data Generation: Generate synthetic datasets based on a flexible prior, simulating a broad range of real-world problems.
Pre-training: A transformer is trained to predict target labels based on masked samples, seeing only the unmasked examples as context.
Inference: Once trained, TabPFN can be used on any real-world dataset without further training, simply using the provided training samples as context to predict unseen labels.
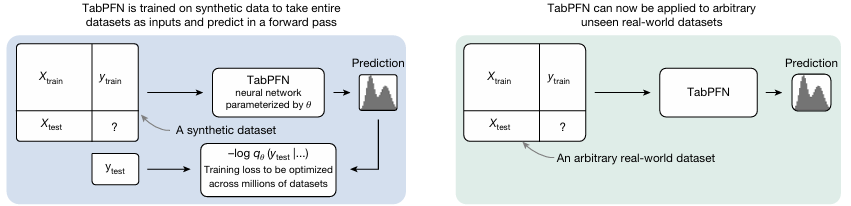
3. Architecture Designed for Tables
TabPFN’s architecture uses two-way attention:
First, each cell attends to other features in its own row (sample-level).
Then, it attends to the same feature across all rows (feature-level).
This clever structure ensures the model is invariant to row/column order, and scales well to larger tables than it was trained on. It's tailor-made for tabular data
4. Synthetic Data Based on Causal Models
Instead of relying on limited or potentially biased real-world data, the authors use synthetic datasets generated from diverse causal structures. These datasets introduce challenges such as non-linearities, different distributions, and noise — preparing TabPFN for real-world robustness.Each training cycle uses around 100 million synthetic datasets, giving the model a vast base of experience. A key advantage is that TabPFN outputs probability distributions, capturing uncertainty in predictions, unlike traditional regression models that return a single point estimate.
5. Results: Quantitative Analysis
TabPFN shows superior predictive performance compared to top-tier models like XGBoost, CatBoost, and Random Forests, even when those are heavily tuned. Especially on classification tasks, it outperforms CatBoost significantly. It also shines in handling dataset characteristics that typically trip up neural networks. In addition to predictions, it supports key foundation model capabilities: data generation, density estimation, fine-tuning, and embedding reuse.
6. Conclusion
TabPFN is a breakthrough in tabular learning — proving that small data doesn’t need small models. By learning across a synthetic universe of tasks, TabPFN becomes a reusable, general-purpose predictor for real-world tabular problems. It moves away from manual model design and tuning toward autonomous learning — a foundation model truly built for tables.